- 1. AI-Driven Strategies Revolutionize Product Formulation, Design & Development in Manufacturing
- 2. AI Empowers Unprecedented Accuracy in Production Quality Inspection
- 3. AI Troubleshooting Advisors: A Manufacturing Lifeline for Predictive Maintenance and Problem-Solving
- 4. Unlock Superior Inventory Management with AI-Driven Demand Forecasting & Prediction
- 5. AI-Driven Adaptive Speed Conveyors: The New Standard for Manufacturing Efficiency
- 6. AI in Last-Mile Delivery: Bridging the Gap Between Factory and Customer Efficiently
- 7. AI-Enabled Loading Docks: The Cornerstone of Smart Warehousing and Efficient Supply Chains
- 8. AI-Powered Pick & Place Robots: The Force Multiplier in Modern Manufacturing
- 9. AI-Driven Smart Forklifts and AGVs: The Backbone of Intelligent Warehousing
- 10. AI’s Role in Minimizing Product Waste and Maximizing Safety in Manufacturing
- 11. AI in Package Lifecycle Tracking: The Keystone for Supply Chain Transparency
- 12. Optimizing Intra-logistics Through AI: A Quantum Leap in Manufacturing Efficiency
- Practical Examples: How Toyota Harnesses AI for Enhanced Manufacturing Efficiency and Innovation
- 3M Amplifies Manufacturing Efficiency with AI-Driven Innovations
- Practical Examples: Samsung Harnesses AI to Propel Chip Manufacturing and Smart Factories
- Practical Examples: PepsiCo Harnesses AI for Manufacturing Excellence and Supply Chain Optimization
- Practical Examples: Amazon Advances Manufacturing and Supply Chain Through Generative AI
Why It Matters
Knowing these AI use cases can elevate your manufacturing processes to new efficiencies.
The Big Picture
Artificial Intelligence (AI) is no longer just a buzzword; it’s an operational necessity. In manufacturing, AI is shaking up the status quo, from design to distribution. Leveraging AI technologies can help companies streamline operations, enhance quality, and drive profitability. In this article, we break down the top 12 AI use cases in manufacturing that are pioneering the industry’s future.
- Product Design & Development
- Quality Inspection
- Troubleshooting
- And much more…
By the Numbers
- The AI in manufacturing market is predicted to reach $16.7 billion by 2026.
- AI can reduce machinery downtime by up to 20%.
- Businesses employing AI for demand forecasting have seen a 10-20% increase in forecast accuracy.
More Details
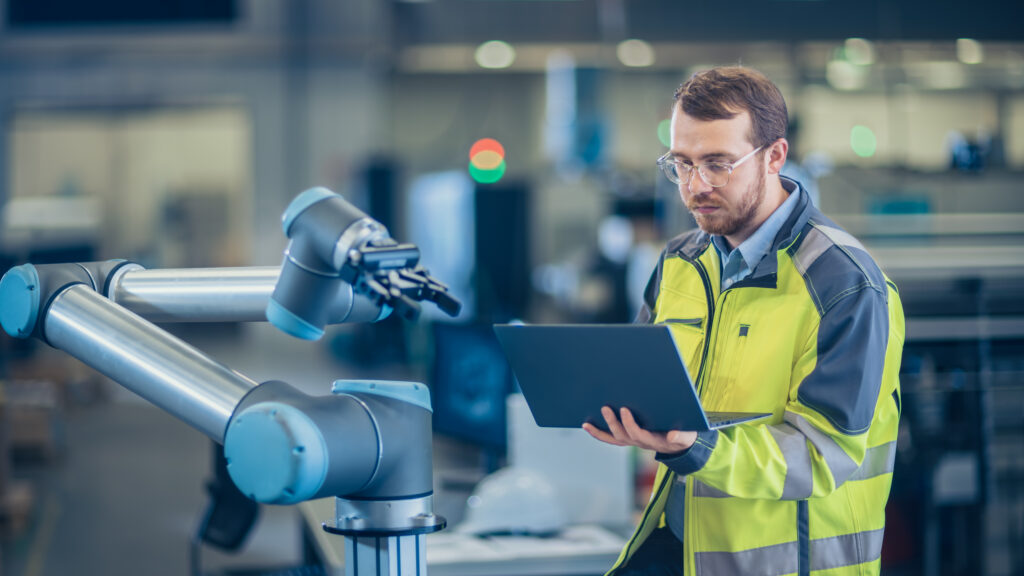
The factory floor isn’t what it used to be. A decade ago, the thought of AI-controlled machines working alongside humans seemed futuristic. Now, it’s a reality impacting multiple facets of manufacturing. Let’s dive into these top 12 applications:
- Product Formulation, Design, and Development: AI algorithms can simulate countless scenarios, optimizing product design and minimizing material waste.
- Production Quality Inspection: Real-time AI analysis ensures that any flaws or inconsistencies are caught immediately.
- Troubleshooting Advisor: AI predictive maintenance reduces downtime by diagnosing machine issues before they become critical.
- Demand Forecasting & Prediction: Gone are the days of guesswork. AI analyzes market trends, consumer behavior, and supply chain variables for precise demand prediction.
- Adaptive Speed Conveyor: Self-adjusting conveyor belts use AI to manage speed according to the production load, reducing energy costs.
- Last-Mile Delivery: AI optimizes routes and ensures that goods reach the customer in the most efficient way.
- Loading Dock Intelligence & Data Capture: Smart docks collect and analyze loading metrics for better warehouse management.
- Robotics Pick & Place: AI-powered robots make the picking and placing process seamless, rapid, and error-free.
- Smart Forklifts/ AGVs/ AMRs: These vehicles navigate autonomously, making warehouse operations faster and safer.
- Product Waste/Spoilage/Safety: AI monitors storage conditions and adjusts them to minimize spoilage.
- Package Lifecycle Tracking: Real-time tracking minimizes lost or delayed shipments.
- Intra-Logistics Simulation & Optimization: AI algorithms analyze the most efficient methods to move goods within a facility, improving operations.
Go Deeper
- Artificial Intelligence in Manufacturing Market Report – MarketsandMarkets
- Predictive Maintenance and Machine Health – IBM
- Demand Forecasting in the Age of Machine Learning – ScienceDirect
- AI in Warehouse Management – McKinsey & Company
And now, let’s dive in each on these use cases.
1. AI-Driven Strategies Revolutionize Product Formulation, Design & Development in Manufacturing
Why It Matters
Harnessing AI in product development can accelerate innovation and dramatically cut costs.
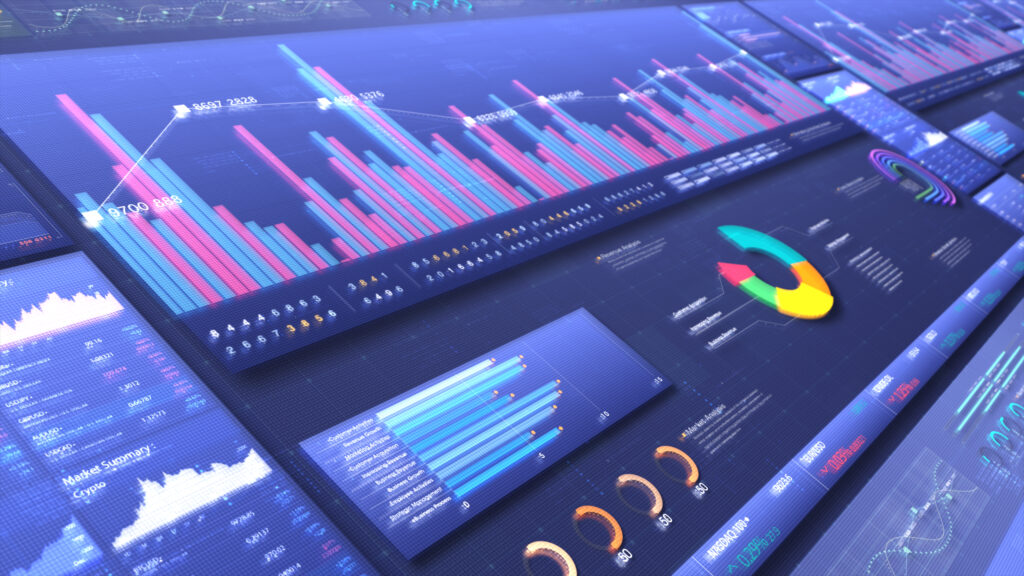
The Big Picture
In the complex world of manufacturing, product formulation, design, and development are intricate processes often subject to high costs and long timelines. AI is turning the tables by providing advanced tools for simulation, optimization, and testing. From conceptualization to the final prototype, AI solutions are making product development smarter, quicker, and more cost-effective.
- Design Optimization
- Materials Testing
- Rapid Prototyping
By the Numbers
- Companies using AI in product development have reduced material costs by up to 15%.
- AI can shorten product development cycles by as much as 20%.
- Simulation software powered by AI can save up to 30% in overall R&D costs.
More Details
AI brings an arsenal of tools to the table in product formulation, design, and development. Here’s a deep dive into how AI is transforming these crucial aspects of manufacturing:
Data-Driven Formulation
AI analyzes complex datasets to recommend ideal product formulations. This method eliminates the trial and error approach, cutting costs and reducing time-to-market.
Dynamic Design Adaptation
Real-time AI algorithms adapt product designs based on multiple variables like market demand or supply chain changes. The end result is a more market-ready product with fewer iterations.
Virtual Prototyping
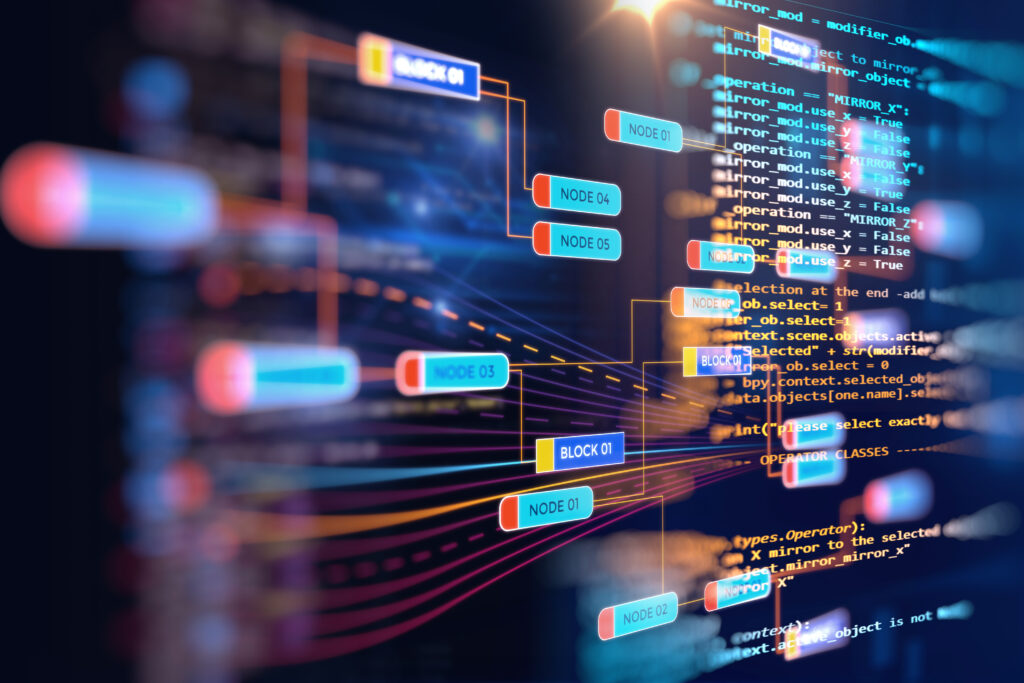
AI models offer a way to virtually prototype and test products in simulated environments. This approach eliminates the need for multiple physical prototypes, further reducing costs and shortening development cycles.
Automated Testing
AI systems can simulate stress tests on materials and components, automating the tedious and time-consuming testing procedures, and ensuring the final product meets all quality standards.
Sustainability and Eco-Friendliness
AI can help manufacturers identify eco-friendly alternatives to materials and processes, thereby promoting sustainability.
Competitive Analysis
Advanced AI models can monitor competitor products and offer insights on features and pricing, helping businesses stay competitive.
Go Deeper
- AI and Product Development: A Game Changer – ResearchGate
- Virtual Prototyping and AI – ScienceDirect
- Automated Testing in Manufacturing – McKinsey & Company
AI’s capabilities in product development are an opportunity to innovate faster, cut costs, and beat the competition. Don’t miss out on leveraging these powerful tools for your manufacturing processes.
2. AI Empowers Unprecedented Accuracy in Production Quality Inspection
Why It Matters
AI-driven quality inspection systems dramatically improve defect detection and compliance, enhancing product reliability.
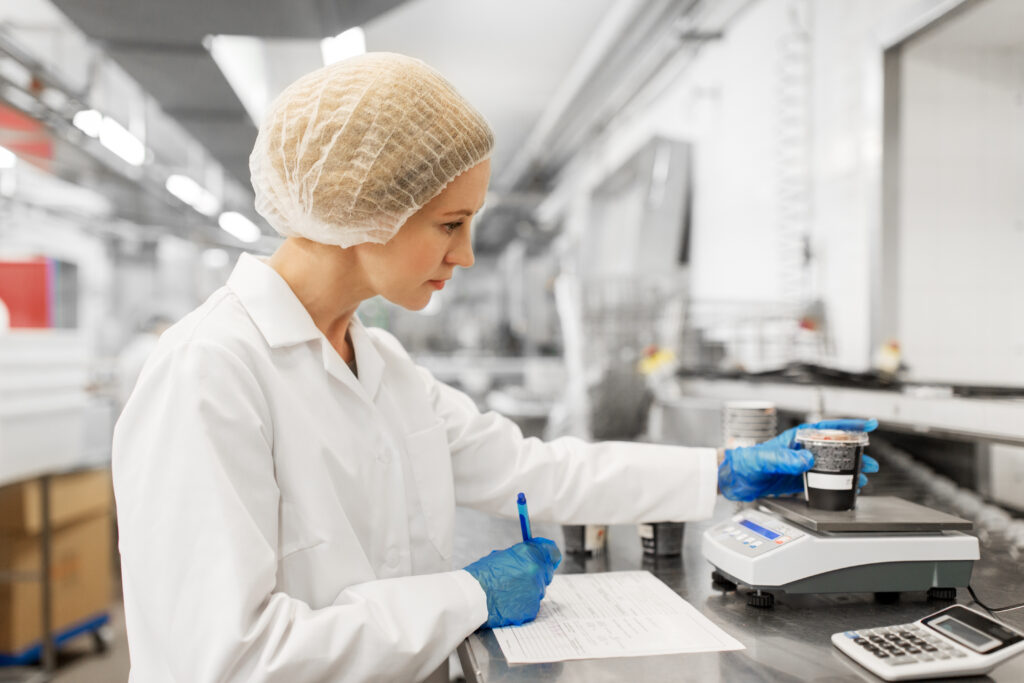
The Big Picture
Quality inspection is a cornerstone of manufacturing. A minor oversight can lead to significant ramifications, including recalls, legal issues, and tarnished brand reputation. AI comes into play here as a game-changer, offering robust, real-time inspection solutions that drastically improve both speed and accuracy.
- Real-time Defect Detection
- Compliance Monitoring
- Continuous Improvement
By the Numbers
- Companies implementing AI for quality inspection have seen up to a 40% reduction in defect rates.
- Automated inspection processes powered by AI can boost throughput by up to 25%.
- AI systems can decrease inspection times by nearly 30%.
More Details
AI technologies are deeply involved in various aspects of quality inspection in manufacturing. Let’s explore how:
Real-time Defect Detection: AI-powered vision systems can scan products on the assembly line in real-time, identifying defects or inconsistencies with unprecedented accuracy.
Compliance Monitoring: AI algorithms assess whether the product complies with all quality standards and regulations, reducing the risk of recalls or legal issues.
Data-Driven Insights: Machine learning models analyze the inspection data to provide actionable insights, enabling the identification and correction of systemic issues in the production line.
Predictive Maintenance: By analyzing machine performance in real-time, AI can predict potential failures that may lead to quality issues, enabling preemptive maintenance.
Statistical Quality Control: AI systems can perform advanced statistical analyses, ensuring that quality control metrics meet or exceed the industry standards.
Worker Training: AI can help train factory workers in best practices for quality inspection, using real-time feedback and interactive simulations.
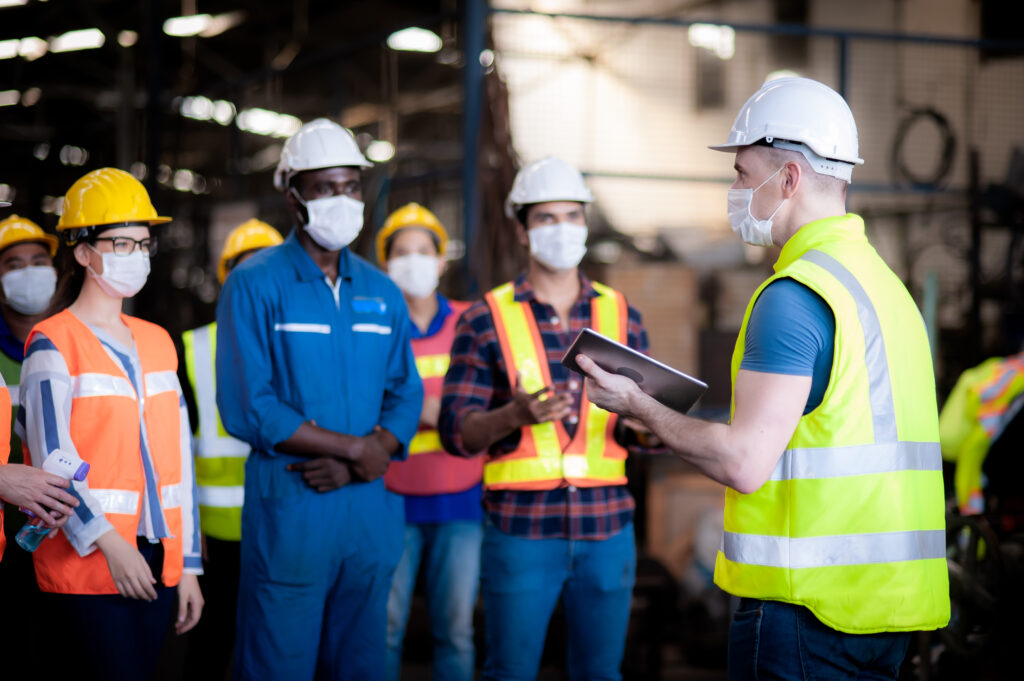
Go Deeper
By implementing AI in your quality inspection processes, you don’t just improve efficiency—you revolutionize your capability to deliver a consistent, high-quality product.
3. AI Troubleshooting Advisors: A Manufacturing Lifeline for Predictive Maintenance and Problem-Solving
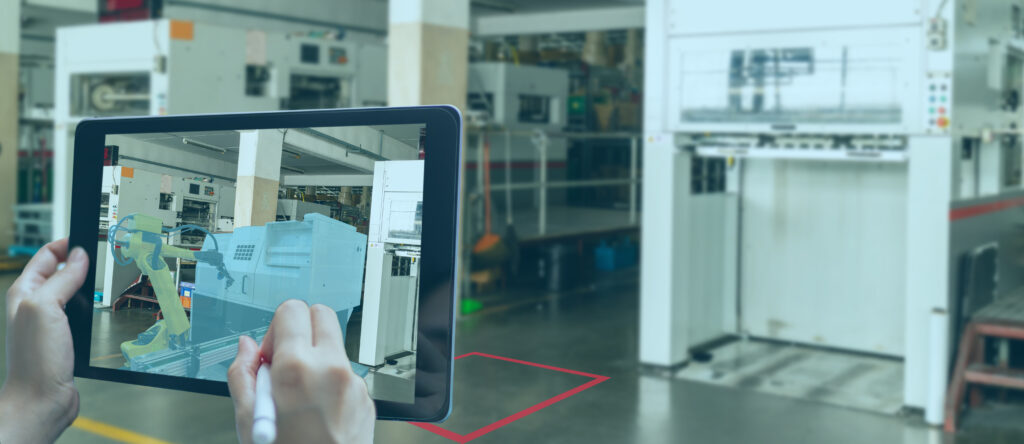
Why It Matters
AI-driven troubleshooting advisors dramatically reduce downtime and operational costs by proactively identifying and solving issues.
The Big Picture
Unplanned downtimes and inefficiencies are the bane of any manufacturing operation. Traditional troubleshooting methods often fall short in timely and effective problem-solving. Enter AI troubleshooting advisors, which employ predictive analytics, machine learning models, and advanced data analytics to foresee, diagnose, and provide solutions for impending issues.
- Predictive Maintenance
- Root Cause Analysis
- Real-Time Problem-Solving
By the Numbers
- AI troubleshooting can reduce machinery downtime by up to 45%.
- Businesses adopting AI troubleshooting advisors save approximately 20% in maintenance costs.
- AI can detect up to 90% of potential equipment failures before they happen.
More Details
AI is profoundly impacting the role of troubleshooting advisors in manufacturing. Here’s how:
Predictive Maintenance: AI algorithms analyze machinery data to predict component failures before they occur, allowing for scheduled maintenance that avoids unplanned downtime.
Root Cause Analysis: AI models can sift through data to determine the root cause of a problem, thereby guiding efficient and effective solutions.
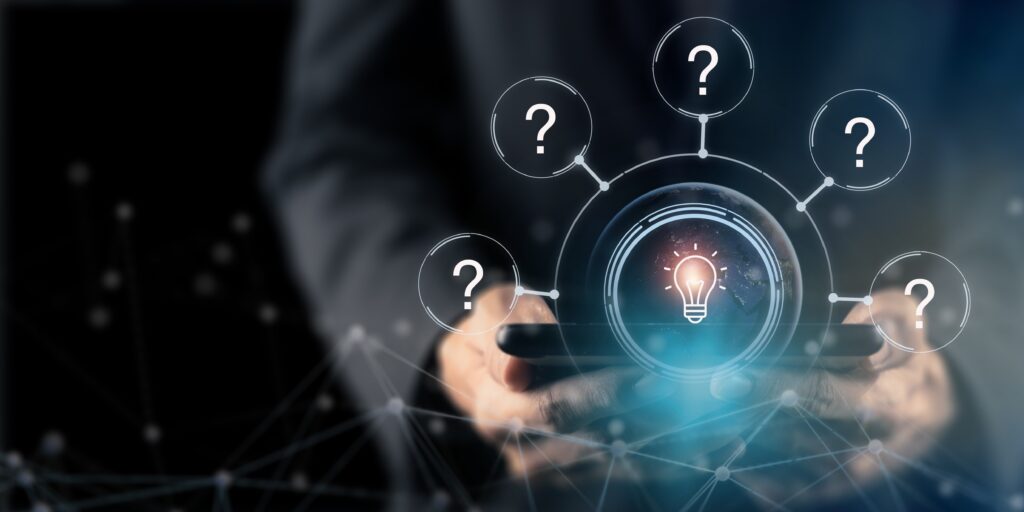
Real-Time Problem-Solving: AI troubleshooting systems offer real-time advice during machine operation, enabling quick fixes without needing to halt production.
Knowledge-Based Systems: AI utilizes vast databases of previous issues and solutions, thereby expediting the troubleshooting process.
Skill Augmentation: AI assists human operators by providing data-driven advice, amplifying their troubleshooting effectiveness.
Resource Optimization: AI helps in allocating maintenance resources where they are needed the most, ensuring cost-effective solutions.
Go Deeper
- Real-time Troubleshooting in Manufacturing – IEEE Xplore
- Efficient Resource Allocation Through AI – McKinsey & Company
Implementing AI as your troubleshooting advisor transforms your approach from reactive to proactive, ensuring smoother operations, reduced costs, and superior productivity.
4. Unlock Superior Inventory Management with AI-Driven Demand Forecasting & Prediction
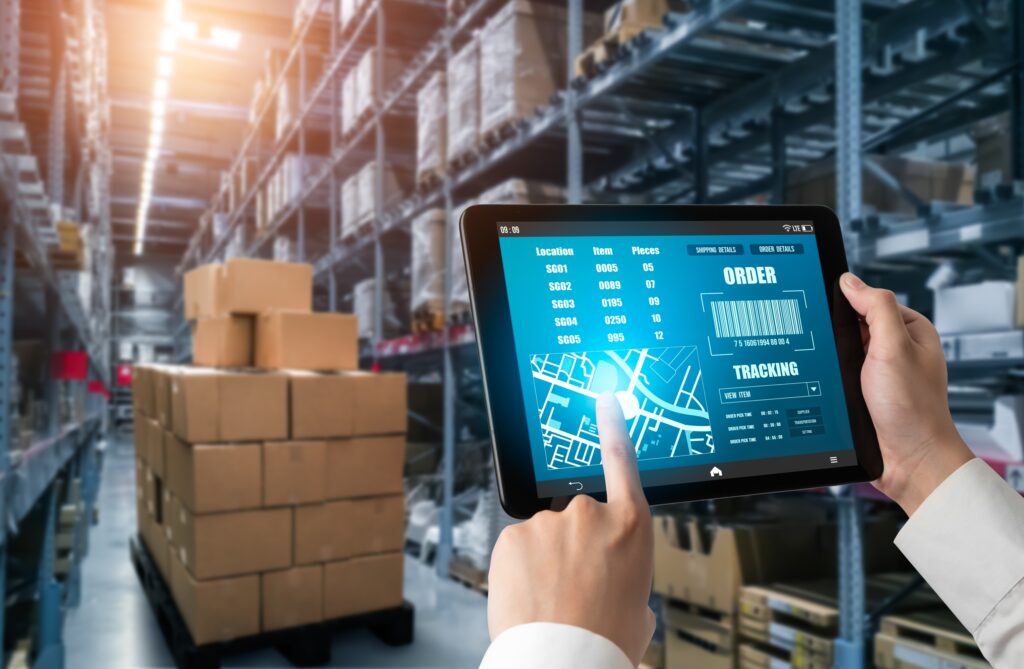
Why It Matters
AI-based demand forecasting directly translates to better inventory management, reduced waste, and maximized profits.
The Big Picture
In an environment driven by customer demands, market fluctuations, and global disruptions, understanding future needs is critical for any manufacturing business. Artificial Intelligence offers a sophisticated, data-driven approach to demand forecasting and prediction, making it easier for businesses to plan production, manage inventory, and allocate resources.
- Seasonal Trend Analysis
- Real-Time Market Adaptation
- Supply Chain Optimization
By the Numbers
- AI-based demand forecasting can increase forecast accuracy by up to 50%.
- Companies utilizing AI in this area have reduced stockouts by 65%.
- AI-driven predictive analysis can boost revenue by 10% through improved stock availability.
More Details
AI serves as a potent tool for demand forecasting and prediction in manufacturing. Let’s delve into the details:
Seasonal Trend Analysis: AI algorithms analyze historical data and seasonal trends to make accurate demand predictions, facilitating better stock management.
Real-Time Market Adaptation: AI models adapt to real-time market changes, offering immediate insights that help businesses adjust their production schedules.
Supply Chain Optimization: With precise demand forecasts, AI helps streamline the supply chain, ensuring timely deliveries and reducing holding costs.
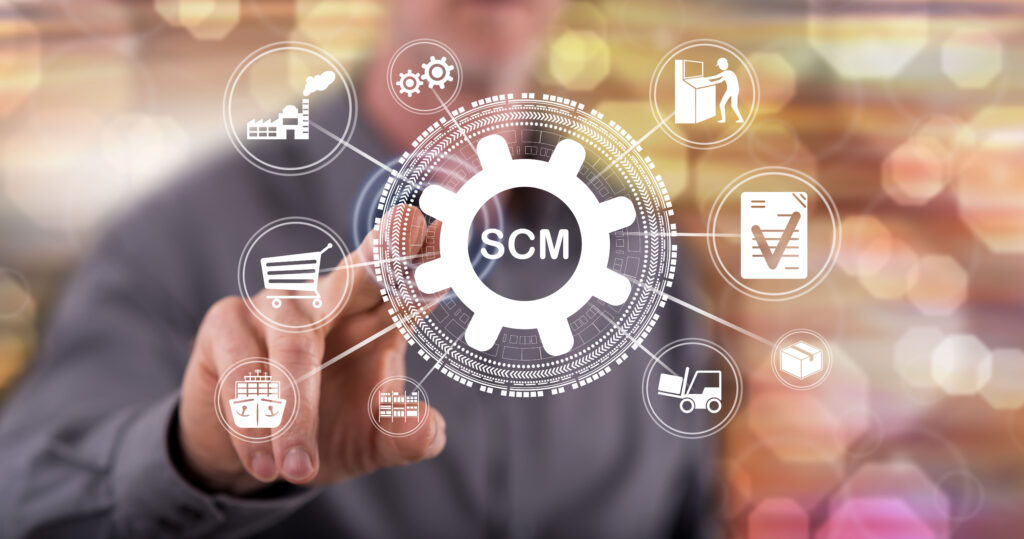
Elasticity Modeling: AI can gauge how external factors such as economic indicators and marketing campaigns impact demand, allowing for smarter planning.
Prescriptive Analytics: Beyond predicting demand, AI also suggests actionable measures to optimize inventory levels and production scheduling.
Multi-Variable Analysis: AI takes into account a wide array of variables like geography, demography, and market behavior for nuanced and comprehensive forecasts.
Go Deeper
- Artificial Intelligence in Demand Forecasting – PLOS ONE
- Supply Chain Optimization Using AI – Deloitte
- Revenue Growth Through AI in Demand Forecasting – Accenture
Harnessing AI for demand forecasting gives you an invaluable edge, ensuring you’re well-prepared to meet market demands while optimizing costs and resources.
5. AI-Driven Adaptive Speed Conveyors: The New Standard for Manufacturing Efficiency
Why It Matters
Adaptive speed conveyors, powered by AI, streamline production lines by autonomously adjusting to real-time demands.
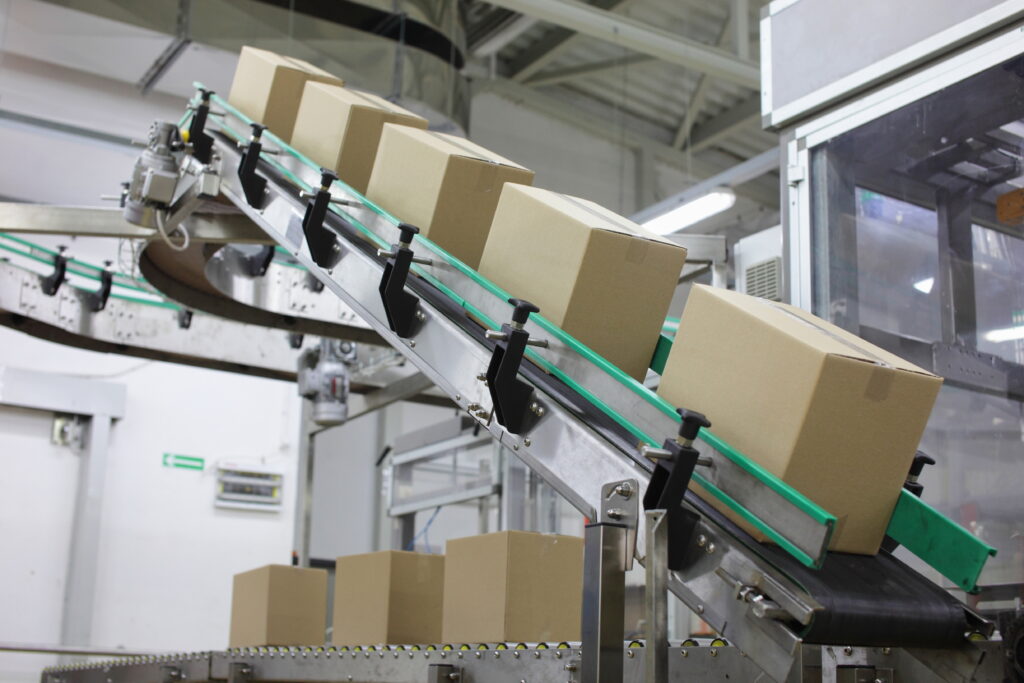
The Big Picture
Manufacturing conveyors have long been the backbone of assembly and production lines. However, traditional conveyors often operate at fixed speeds, leading to inefficiencies and bottlenecks. AI-driven adaptive speed conveyors are rewriting the rule book by adjusting their speed and operations in real-time to match production needs.
- Dynamic Speed Adjustment
- Energy Efficiency
- Improved Throughput
By the Numbers
- Adaptive speed conveyors can increase production throughput by up to 35%.
- Energy consumption can be reduced by approximately 25% with AI-based adaptive systems.
- Downtime is decreased by an estimated 20% when using adaptive speed conveyors.
More Details
AI’s role in adaptive speed conveyors is multifaceted, adding layers of efficiency to manufacturing operations:
Dynamic Speed Adjustment: AI algorithms analyze real-time production data to autonomously adjust conveyor speeds, ensuring optimal productivity and reducing bottlenecks.
Energy Efficiency: By operating only at necessary speeds, AI-driven conveyors conserve energy, contributing to sustainability goals.
Sensor Integration: Sensors feed data into the AI system, which then makes real-time adjustments to avoid product collisions and ensure smooth transport.
Predictive Maintenance: The AI system can forecast wear and tear, scheduling maintenance activities that avoid production interruptions.
Workflow Synchronization: Adaptive speed conveyors, directed by AI, can synchronize with other machinery for a harmonious, efficient production flow.
Data-Driven Insights: Detailed operational data is analyzed for long-term improvements in conveyor system design and performance optimization.
Go Deeper
- AI and Conveyor Systems: A Perfect Match – IEEE Xplore
- Adaptive Conveyors in Modern Manufacturing – ResearchGate
- Industrial Automation: AI’s Growing Role – McKinsey & Company
Adopting AI-driven adaptive speed conveyors is not an incremental upgrade; it’s a transformational leap in manufacturing efficiency and effectiveness.
6. AI in Last-Mile Delivery: Bridging the Gap Between Factory and Customer Efficiently
Why It Matters
AI-driven last-mile delivery solutions drastically optimize the most complex, cost-intensive segment of the supply chain.
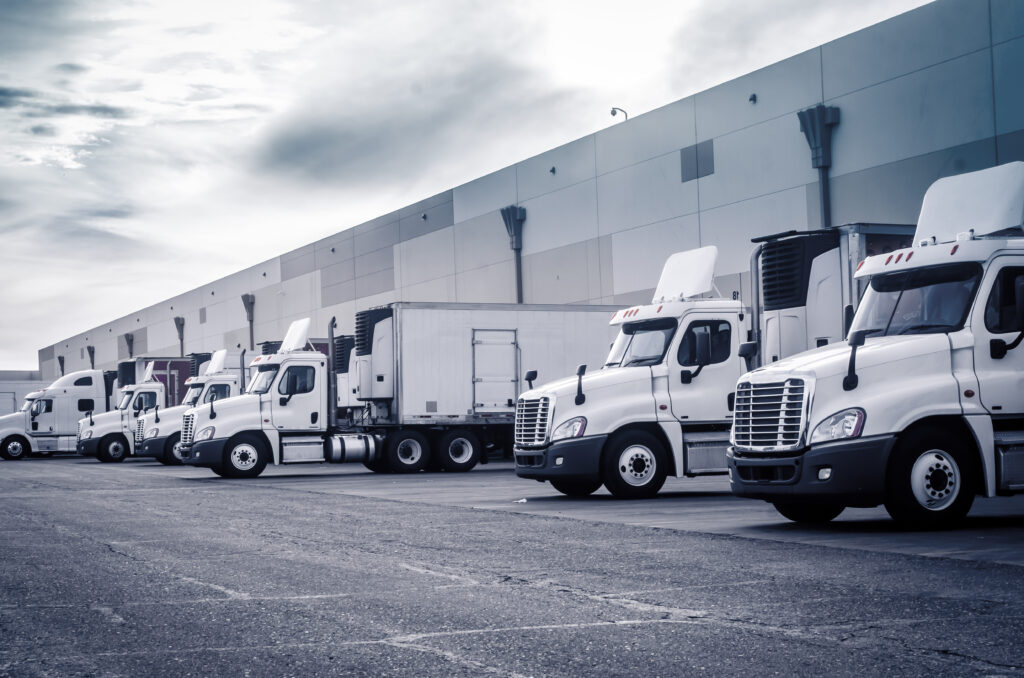
The Big Picture
The last-mile—delivering a product from the local distribution center to the customer—is often fraught with inefficiencies, delays, and extra costs. AI steps in to alleviate these issues, transforming last-mile delivery into a more efficient, cost-effective, and customer-centric operation.
- Dynamic Routing
- Real-Time Adjustments
- Customer Experience Enhancement
By the Numbers
- AI can reduce last-mile delivery costs by up to 30%.
- 40% improvement in on-time deliveries has been observed with AI deployment.
- Customer satisfaction scores can improve by approximately 15% through AI-enhanced last-mile solutions.
More Details
AI is making significant strides in optimizing the last-mile delivery for manufacturers:
Dynamic Routing: AI algorithms evaluate multiple factors, such as traffic and weather, to determine the most efficient delivery routes.
Real-Time Adjustments: AI systems adapt dynamically to unforeseen circumstances, rerouting deliveries in real-time to mitigate delays.
Inventory Matching: By analyzing real-time inventory levels and demand, AI ensures the right products are ready for dispatch, reducing stockouts and overstocking.
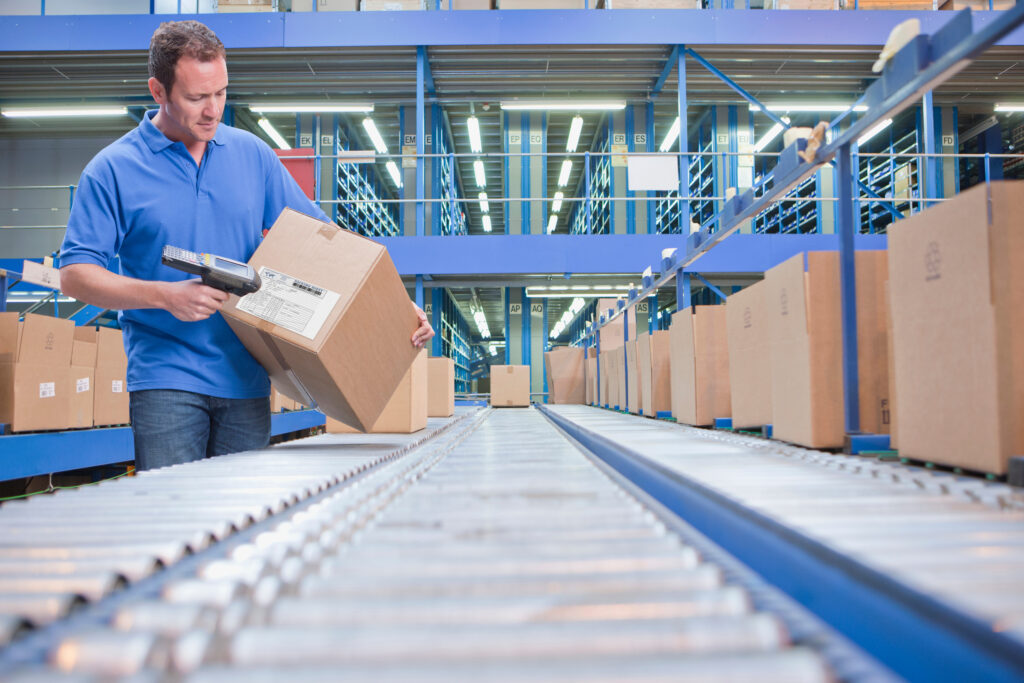
Customer Experience Enhancement: AI tools like chatbots keep customers informed about delivery status, while predictive analytics forecast delivery windows with remarkable accuracy.
Sustainable Operations: AI can optimize delivery routes and vehicle loads to reduce carbon emissions, contributing to sustainability objectives.
Analytics and Insights: Post-delivery data is analyzed to refine future operations, improving efficiency and customer satisfaction over time.
Go Deeper
- Last-Mile Delivery Models: Optimized by AI – ScienceDirect
- Enhancing Customer Experience in Last-Mile Delivery – Deloitte
- Sustainability in Last-Mile Logistics – Accenture
By integrating AI into last-mile delivery, manufacturers can vastly improve efficiency, reduce costs, and enhance the overall customer experience.
7. AI-Enabled Loading Docks: The Cornerstone of Smart Warehousing and Efficient Supply Chains
Why It Matters
Intelligent loading docks driven by AI significantly enhance data capture, boosting supply chain efficiency and reducing operational risks.
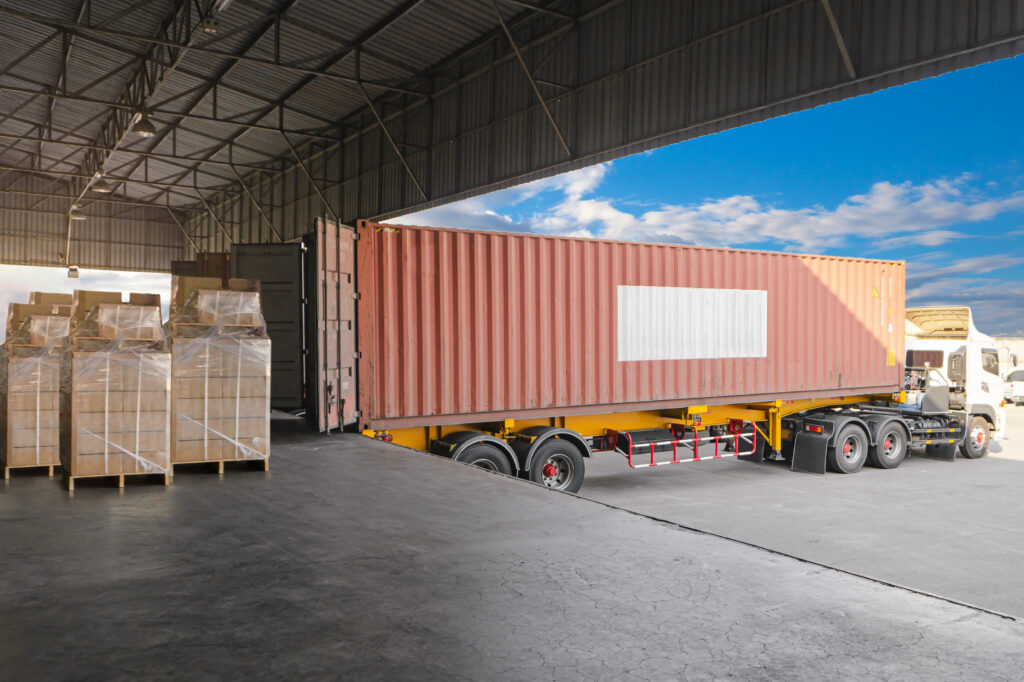
The Big Picture
Loading docks are critical touchpoints in any supply chain, yet they are often riddled with inefficiencies, data gaps, and safety risks. Artificial Intelligence transforms these docks into intelligent hubs, automating data capture and optimizing various processes.
- Automated Data Capture
- Risk Mitigation
- Real-time Inventory Management
By the Numbers
- AI can reduce loading and unloading times by up to 40%.
- Error rates in inventory recording can be cut by almost 30% through AI-driven data capture.
- Implementation of AI in loading docks can improve safety measures by around 20%.
More Details
AI adds several layers of innovation and efficiency to loading dock operations:
Automated Data Capture: Smart sensors and cameras record critical data such as shipment contents, dimensions, and condition, eliminating human errors.
Risk Mitigation: AI algorithms analyze captured data to identify risks like unsafe stacking or hazardous materials, enabling timely corrective actions.
Real-time Inventory Management: AI integrates captured data with existing inventory systems for real-time tracking and optimization.
Time Efficiency: Predictive algorithms can foresee loading and unloading times based on historical data, helping in better dock scheduling.
Analytics and Reporting: AI-driven analytics tools process the captured data to generate actionable insights for performance improvement.
Supply Chain Synchronization: Data captured at the loading dock can be integrated into the wider supply chain analytics, streamlining operations from manufacturing to delivery.
Go Deeper
- Automating Warehousing Operations – IEEE Xplore
- Risk Management in Supply Chains – McKinsey & Company
- IoT in Supply Chain Management – Deloitte
Investing in AI-enabled loading docks is an imperative for modern manufacturers aiming for a data-driven, risk-averse, and highly efficient supply chain.
8. AI-Powered Pick & Place Robots: The Force Multiplier in Modern Manufacturing
Why It Matters
AI-driven pick & place robotics drastically improve both speed and accuracy in manufacturing, elevating operational efficiency.
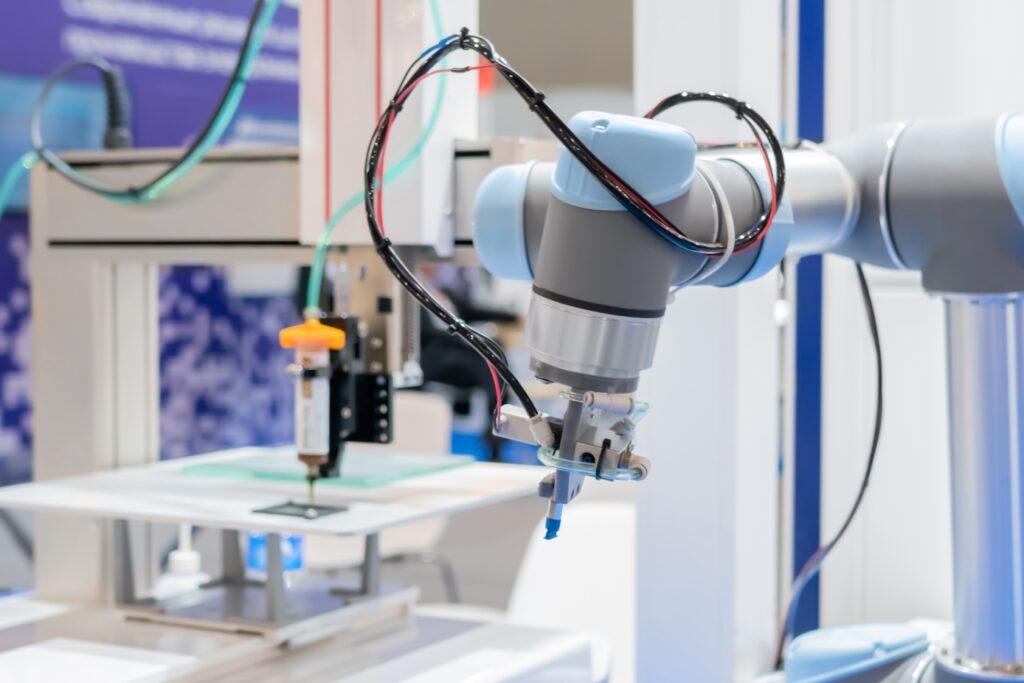
The Big Picture
Robotic arms for pick & place tasks have been around, but AI elevates their utility to new heights. By incorporating machine learning algorithms and advanced sensors, these robots can adapt to varying scenarios, handling complex tasks with minimal human intervention.
- Adaptive Learning
- Precision & Speed
- Collaborative Operations
By the Numbers
- Efficiency gains of up to 50% are seen in operations utilizing AI-enabled pick & place robots.
- Human error is reduced by approximately 35%.
- AI-driven systems can achieve a picking accuracy rate of almost 99%.
More Details
Here’s how AI is revolutionizing pick & place robotics in manufacturing:
Adaptive Learning: AI algorithms allow the robot to learn from its environment and improve its picking strategies over time.
Precision & Speed: Advanced sensors and machine vision contribute to the robot’s ability to pick items with high precision and speed, regardless of variations in size, shape, or orientation.
Collaborative Operations: AI-driven robots can work alongside human operators safely and efficiently, thanks to real-time adaptive algorithms.
Fault Tolerance: Machine learning helps the robot adapt to minor deviations and malfunctions, ensuring continuous operations.
Inventory Optimization: AI can integrate the pick & place data into inventory systems, optimizing stock levels and minimizing waste.
Real-time Analytics: Data collected by the robots can be analyzed for real-time insights into operational efficiency, facilitating timely decision-making.
Go Deeper
- Machine Learning for Robotics – IEEE Xplore
- AI in Industrial Automation – McKinsey & Company
- Collaborative Robots in Industry – Accenture
Adopting AI-enabled pick & place robots is a strategic move for manufacturers seeking to amplify efficiency, accuracy, and overall productivity.
9. AI-Driven Smart Forklifts and AGVs: The Backbone of Intelligent Warehousing
Why It Matters
Leveraging AI in smart forklifts, AGVs, and AMRs transforms warehousing operations, achieving unprecedented levels of efficiency and safety.
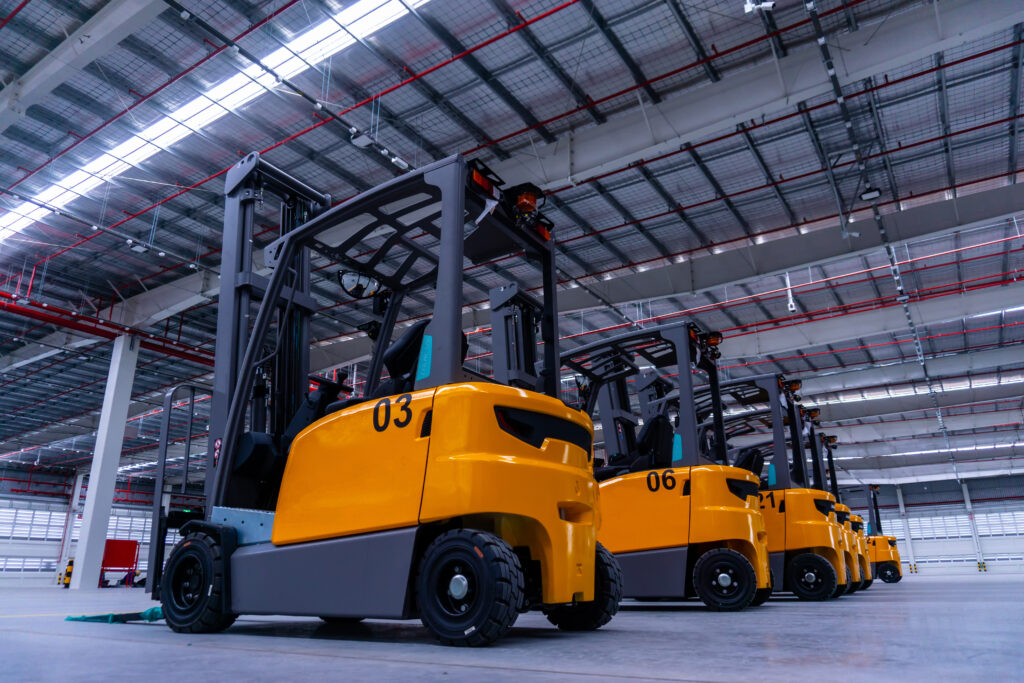
The Big Picture
Smart forklifts, Automated Guided Vehicles (AGVs), and Autonomous Mobile Robots (AMRs) equipped with AI capabilities have emerged as game-changers in warehousing and intra-logistics. These AI-driven machines not only streamline tasks but also make operations safer and more reliable.
- Path Optimization
- Load Balancing
- Safety Enhancements
By the Numbers
- AI can increase warehouse space utilization by up to 25%.
- AI-enabled smart forklifts can decrease the risk of accidents by about 30%.
- AGVs and AMRs can improve operational efficiency by a staggering 50%.
More Details
AI brings several layers of advantages to smart forklifts, AGVs, and AMRs:
Path Optimization: AI algorithms continuously analyze warehouse layouts and traffic to choose the most efficient routes for these machines.
Load Balancing: AI ensures optimal distribution of tasks among multiple forklifts or AGVs, avoiding bottlenecks and maximizing productivity.
Safety Enhancements: Sophisticated sensors and machine learning models allow for early hazard detection, thereby reducing the likelihood of accidents.
Adaptive Operations: These AI-powered machines can adapt to changes in warehouse inventory layout or task priority, requiring minimal manual reconfiguration.
Energy Efficiency: AI-driven analytics can also optimize the energy consumption of these machines, contributing to sustainability goals.
Real-time Monitoring: AI allows for the constant tracking and monitoring of these vehicles, facilitating immediate adjustments and long-term improvements.
Go Deeper
- Robotics and AI in Warehouse Management – IEEE Xplore
- Creating a Safer Workplace in Logistics – McKinsey & Company
- Sustainable Warehousing – Deloitte
Manufacturers stand to gain significantly in operational efficiency and safety by integrating AI into their warehousing machines like smart forklifts, AGVs, and AMRs.
10. AI’s Role in Minimizing Product Waste and Maximizing Safety in Manufacturing
Why It Matters
Integrating AI into manufacturing operations can substantially mitigate product waste, spoilage, and safety concerns, directly impacting profitability and sustainability.
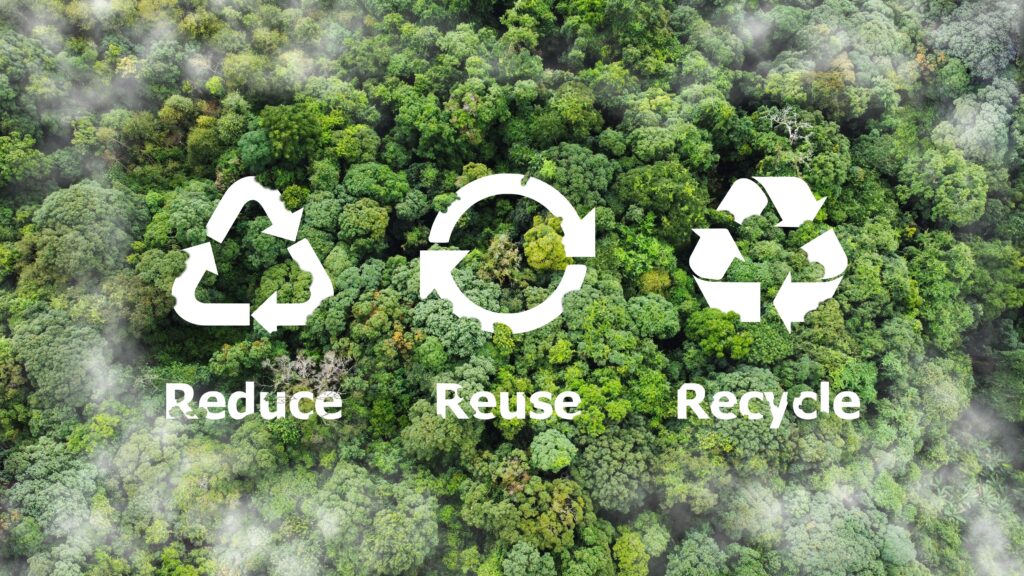
The Big Picture
Traditional methods often fail to adequately address the complexities associated with waste, spoilage, and safety in manufacturing. AI opens up new avenues for proactive management of these issues through real-time monitoring, predictive analytics, and automated interventions.
- Real-time Monitoring
- Predictive Analytics
- Automated Interventions
By the Numbers
- Up to 20% reduction in product waste when leveraging AI-based systems.
- AI can predict equipment failures with 85% accuracy, reducing spoilage risks.
- Safety incidents can drop by nearly 30% with the use of AI-driven safety measures.
More Details
Here’s how AI is shaping waste, spoilage, and safety management in manufacturing:
Real-time Monitoring: AI-enabled sensors can continuously track production metrics and environmental conditions, identifying waste and spoilage risks in real-time.
Predictive Analytics: Machine learning models can forecast equipment malfunctions and process inefficiencies that may result in waste or spoilage, allowing for preventive action.
Automated Interventions: AI systems can automatically adjust machine settings or halt operations if they detect conditions that might lead to waste, spoilage, or unsafe situations.
Quality Control: Advanced AI algorithms can scan through product batches to identify and segregate defective items, significantly reducing waste.
Sustainability: AI’s role in minimizing waste contributes to more sustainable operations, which is increasingly important for regulatory compliance and corporate responsibility.
Safety Protocols: AI can automate safety checks and trigger alerts, enhancing the overall safety landscape of the manufacturing floor.
Go Deeper
- Predictive Analytics in Manufacturing – IEEE Xplore
- Workplace Safety Enhancement through AI – McKinsey & Company
- Compliance and Risk Management – Deloitte
The application of AI for minimizing waste, spoilage, and enhancing safety can revolutionize manufacturing, making it not just more profitable, but also more responsible.
11. AI in Package Lifecycle Tracking: The Keystone for Supply Chain Transparency
Why It Matters
AI-driven package lifecycle tracking dramatically boosts visibility, accountability, and efficiency across the entire supply chain.
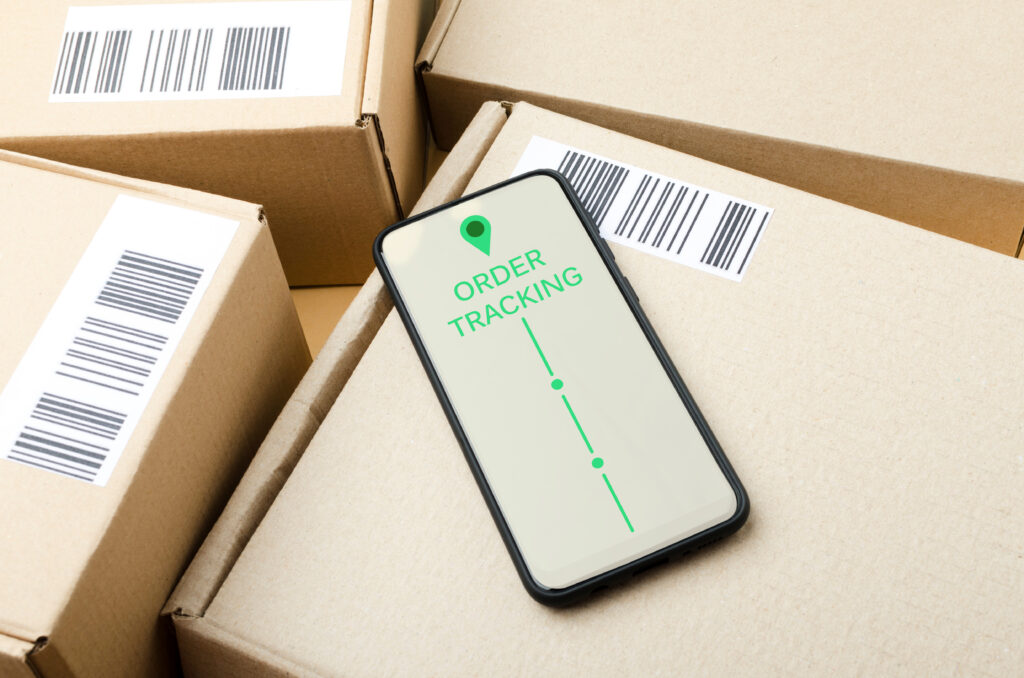
The Big Picture
AI transforms package lifecycle tracking by offering real-time data and predictive insights, enabling stakeholders to make informed decisions at every step of the supply chain. Advanced analytics and machine learning models facilitate precise tracking of packages from production to delivery.
- Real-Time Visibility
- Predictive Milestones
- Risk Mitigation
By the Numbers
- 98% tracking accuracy achieved with AI-based lifecycle tracking systems.
- Companies report a 25% reduction in lost or misplaced items within the supply chain.
- Up to 40% improvement in delivery times using AI algorithms for route optimization.
More Details
The capabilities AI brings to package lifecycle tracking are game-changing:
Real-Time Visibility: AI algorithms provide real-time tracking data, making it easier to locate packages at any point in the supply chain.
Predictive Milestones: Machine learning can forecast potential delays and suggest alternate routes or strategies, minimizing disruptions.
Risk Mitigation: By analyzing historical data, AI can identify high-risk scenarios, such as theft or spoilage, and suggest preventive measures.
Compliance Tracking: AI systems can automatically flag any non-compliance issues, ensuring that all regulations are followed.
Cost Efficiency: Optimized route planning and reduced losses contribute to cost savings, enhancing the overall profitability of supply chain operations.
Customer Satisfaction: Real-time tracking and accurate delivery estimations significantly enhance customer experience and trust.
Go Deeper
- Real-Time Data in Logistics – IEEE Xplore
- Improving Supply Chain Efficiency – McKinsey & Company
- AI in Customer Experience Management – Deloitte
AI-powered package lifecycle tracking is becoming an indispensable tool for modern supply chains, offering unmatched efficiency, compliance, and customer satisfaction.
12. Optimizing Intra-logistics Through AI: A Quantum Leap in Manufacturing Efficiency
Why It Matters
AI-driven intra-logistics simulation and optimization are integral for achieving unprecedented operational efficiency and resource allocation in manufacturing.
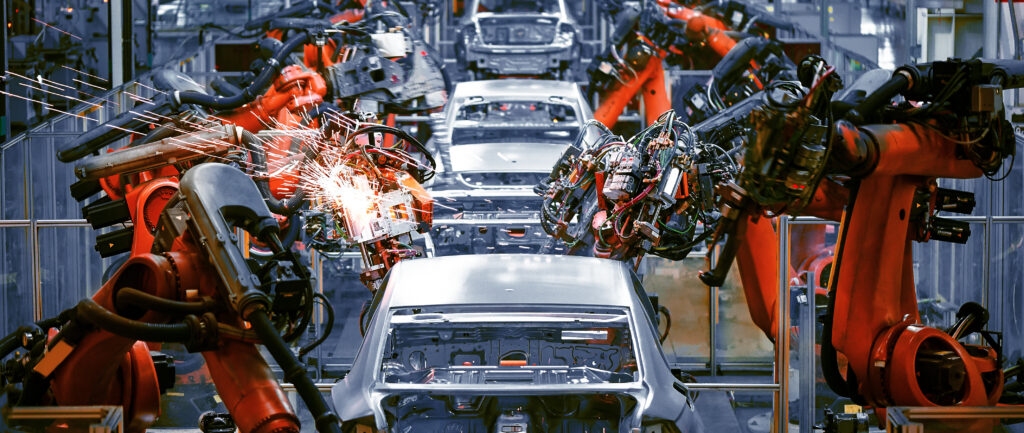
The Big Picture
Intra-logistics, the movement and flow of materials within a manufacturing facility, has seen substantial improvement through the application of AI. Advanced algorithms simulate numerous scenarios, optimizing pathways, reducing congestion, and aiding in effective resource allocation.
- Scenario Simulation
- Dynamic Pathways
- Resource Allocation
By the Numbers
- Up to a 35% reduction in intra-logistics operational costs with AI.
- A 40% improvement in resource utilization by employing AI simulations.
- Decrease in lead times by an average of 25% through optimized logistics.
More Details
AI brings groundbreaking capabilities to intra-logistics in manufacturing:
Scenario Simulation: AI-based systems can run multiple scenarios to predict the most effective logistical routes, considering variables such as time, resource availability, and existing obstructions.
Dynamic Pathways: AI algorithms adapt in real-time, changing routes dynamically based on real-time data to prevent congestion and delays.
Resource Allocation: Through predictive analytics, AI helps allocate resources optimally, thus preventing bottlenecks and ensuring smooth operations.
Time Efficiency: AI-based optimization minimizes lead times, allowing for quicker processing and shipment of orders.
Cost-Effectiveness: By optimizing routes and resource utilization, AI dramatically reduces operational costs involved in intra-logistics.
Scalability: AI-driven solutions are scalable, allowing for easy adjustments as a manufacturing facility grows or changes its operations.
Go Deeper
- Dynamic Routing for Logistics – IEEE Xplore
- Optimizing Factory Operations – McKinsey & Company
- AI-Driven Scalability in Manufacturing – Deloitte
AI’s application in intra-logistics simulation and optimization paves the way for manufacturers to achieve unparalleled operational efficiency and strategic resource allocation.
Practical Examples: How Toyota Harnesses AI for Enhanced Manufacturing Efficiency and Innovation
Why it matters
Toyota’s AI deployment in manufacturing underscores a broader industry shift towards smart factories, driving both operational excellence and innovation.
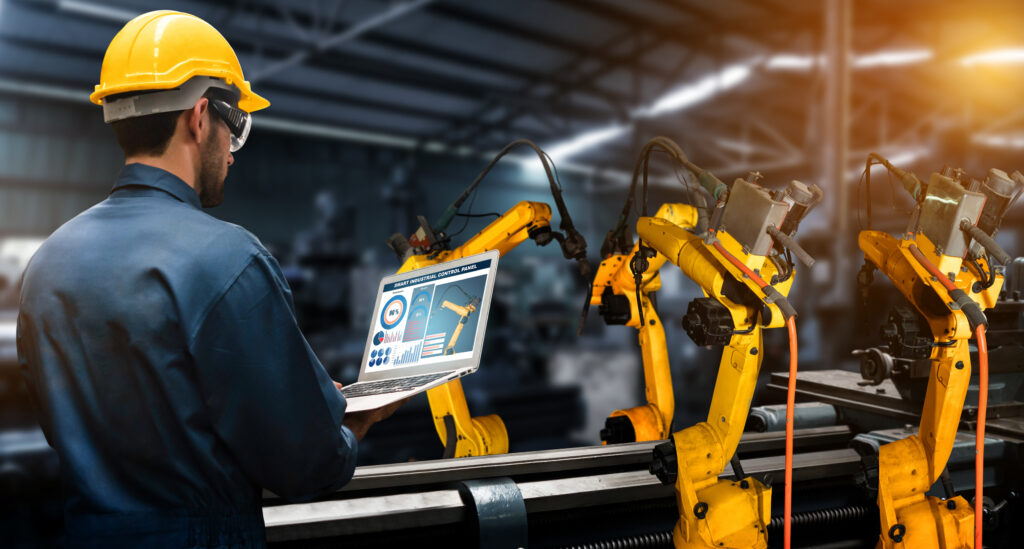
The Big Picture
Toyota has been making significant strides in integrating Artificial Intelligence (AI) into its manufacturing processes to bolster efficiency, innovation, and sustainability. Here are some notable implementations:
- Computer Vision Systems:
- Partnering with Invisible AI, Toyota deployed an AI computer vision system in factories to enhance operational efficiency1.
- Predictive Analytics:
- AI-driven predictive analytics are utilized for foreseeing production abnormalities, exemplified in Toyota’s collaboration with Siemens in the die casting process2.
- Robotics and Automation:
- AI, alongside robotics, is propelling Toyota towards Manufacturing 4.0, with technologies driving automation and real-time data analytics3.
- Generative AI Techniques:
- In 2023, Toyota Research Institute unveiled a generative AI technique aiming to amplify vehicle design processes4.
- Autonomous and Automated Vehicles:
- Significant investments have been made in R&D for autonomous and automated vehicles leveraging AI, machine learning, and computer vision5.
By the Numbers
- The advent of AI in manufacturing is predicted to create a value between $1.2 to $2 trillion, illustrating the immense potential for companies like Toyota5.
- AI adoption in manufacturing is showcasing a 10% increase in output in specific case studies, hinting at substantial productivity gains5.
More Details
Toyota’s journey towards smart manufacturing through AI is emblematic of the broader industrial transition into the digital era. The deployment of computer vision systems in collaboration with Invisible AI underscores the potential of AI in monitoring and enhancing factory operations. Furthermore, predictive analytics, powered by AI, are enabling Toyota to foresee and mitigate production abnormalities, optimizing the manufacturing process and minimizing downtime.
The partnership with Siemens in integrating AI into the die casting process is a testament to the collaborative nature of industrial AI adoption. Moreover, Toyota’s emphasis on robotics and automation, driven by AI technologies, is setting the stage for enhanced operational efficiency and real-time data analytics.
Generative AI techniques introduced by the Toyota Research Institute are not only boosting the design process but are indicative of the broader scope of AI in fostering innovation within manufacturing. The significant investments in autonomous and automated vehicles underscore Toyota’s forward-thinking approach in leveraging AI for both manufacturing and product innovation.
Go Deeper
- Toyota and Invisible AI Collaboration – Explore the partnership between Toyota and Invisible AI in deploying AI computer vision systems in factories.
- Siemens and Toyota Collaboration – Delve into the collaborative effort between Siemens and Toyota in integrating AI into the die casting process.
- Toyota Research Institute’s Generative AI – Understand the generative AI techniques introduced by the Toyota Research Institute for amplifying vehicle design processes.
- Artificial Intelligence at Toyota – Gain insights into Toyota’s broader AI strategy and its impact on autonomous and automated vehicles.
These resources provide a more granulated understanding of Toyota’s AI initiatives within manufacturing and beyond, illustrating the transformative potential of AI in modern industrial operations.
3M Amplifies Manufacturing Efficiency with AI-Driven Innovations
Why it matters
3M’s utilization of AI in manufacturing showcases the breadth of AI’s application in optimizing operations, enhancing product quality, and driving innovation.
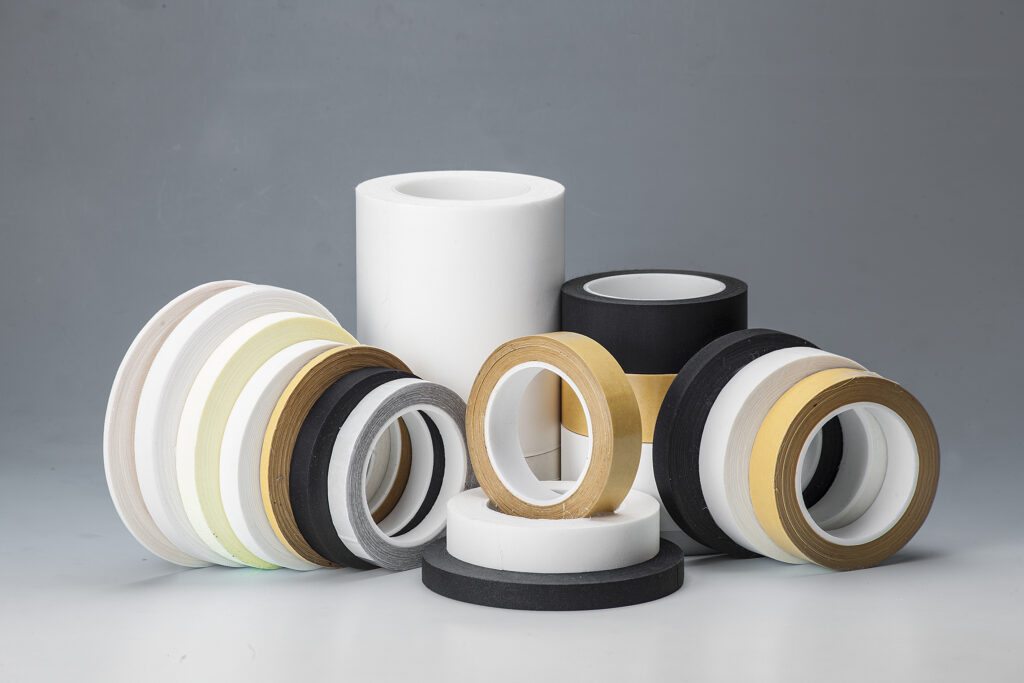
The Big Picture
- Predictive Analytics: AI is employed to optimize manufacturing, supply chain operations, and improve production quality.
- Process Enhancement: AI aids in developing and manufacturing new materials and processes through advanced algorithms.
- Real-Time Optimization: Utilization of real-time data for ensuring safety and optimizing equipment efficiency in manufacturing settings.
More details
Discover how 3M implements AI across its divisions to solve diverse challenges and drive operational excellence here.
Practical Examples: Samsung Harnesses AI to Propel Chip Manufacturing and Smart Factories
Why it matters
Samsung’s adoption of AI technologies in manufacturing underscores a broader industry shift towards data-driven, automated, and efficient production systems.
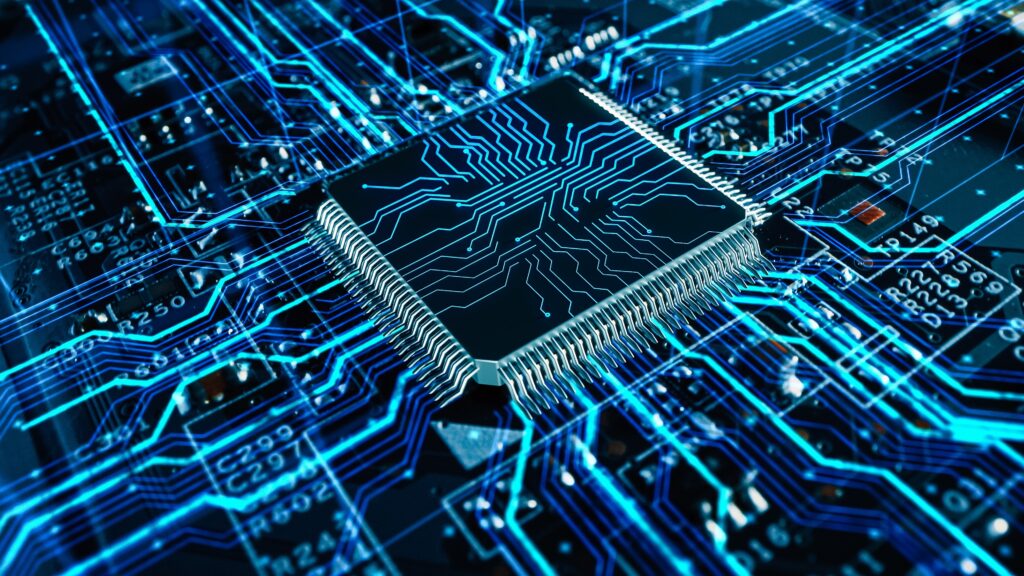
The Big Picture
- Chip Manufacturing Transformation:
- Boosting productivity and refining product quality with AI and Big Data to compete with major foundry rival, Taiwan Semiconductor Manufacturing Co.
- Employing AI across various stages including DRAM design automation, chip material development, foundry yield improvement, mass production, and chip packaging.
- Utilizing AI to address challenges arising from ultra-fine chipmaking processes like transistor interference and leakage current.
- Foundry Technology Innovations:
- Advancing semiconductor technology to cater to customer needs in the AI era, showcased at the Samsung Foundry Forum 2023.
- Unveiling plans for mass production of 2nm process targeting mobile applications (2025), HPC (2026), and automotive (2027) sectors, alongside a new “Multi-Die Integration (MDI) Alliance” for next-gen packaging technology.
- Smart Manufacturing and AI Forums:
- Hosting forums to showcase the latest in AI and computer engineering, reflecting a continuous endeavor towards integrating AI in manufacturing and beyond.
- AI in Product Optimization and Other Domains:
- Deploying AI-based systems like DSO.ai for optimizing mobile designs, indicating a wider scope of AI applications beyond manufacturing.
- Venturing into Food AI and Vision AI technologies for enhanced user experiences, demonstrating the diversification of AI applications.
By The Numbers
- 12% Increase in performance with Samsung’s 2nm process compared to its 3nm process.
- 25% Increase in power efficiency with the 2nm process.
- 5% Decrease in area with the 2nm process, showcasing the potential efficiency gains from AI and advanced manufacturing technologies.
More Details
Uncover a deeper understanding of Samsung’s AI-driven manufacturing innovations here and here.
Go Deeper
- Generative AI in Manufacturing: Explore how generative AI and cutting-edge applications are reshaping manufacturing demands for smarter and more powerful computing, in line with Samsung’s focus on smart manufacturing.
- Global AI Forums and Collaborations: Delve into global forums like Samsung AI Forum 2023, where industry leaders share the latest advancements in AI and computer engineering.
- AI in Product Development: Discover how AI is utilized in optimizing product designs and creating enhanced user experiences across different domains.
Practical Examples: PepsiCo Harnesses AI for Manufacturing Excellence and Supply Chain Optimization
Why it matters
PepsiCo’s strategic deployment of AI in manufacturing and supply chain processes underscores a data-driven approach to enhance operational efficiency, consumer engagement, and product innovation.
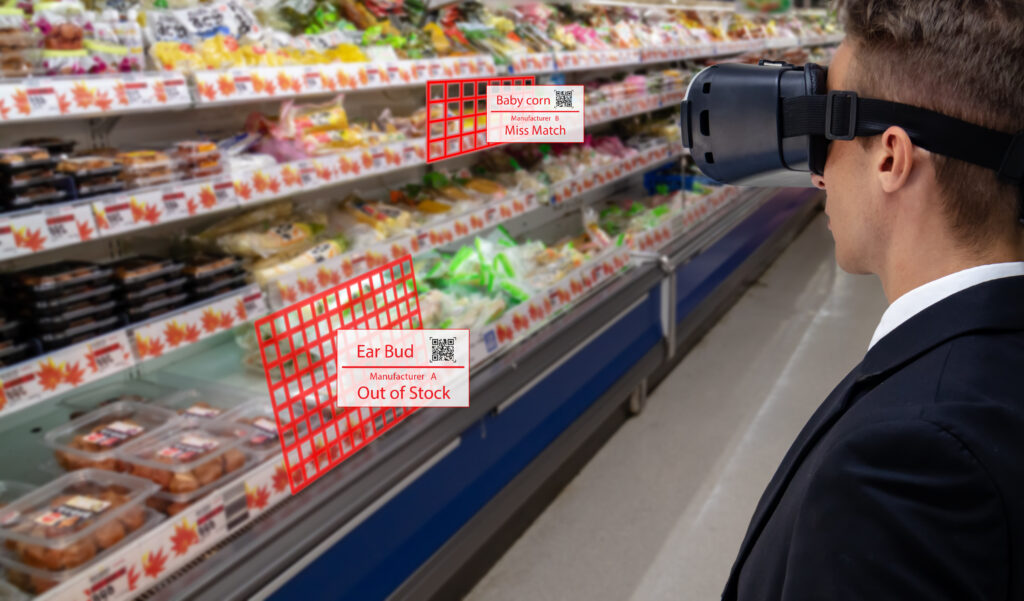
The Big Picture
- Advanced Machine Vision:
- Utilization of machine vision technology from KoiReader Technologies to boost efficiency and accuracy in distribution processes.
- Collaboration with Academic Institutions:
- Partnership with Stanford Institute for Human-Centered Artificial Intelligence focusing on supply chains, forecasting, and smart manufacturing to optimize daily operations throughout the supply chain, and better predict inventory levels and demand patterns.
- Incorporation of AI in Daily Operations:
- AI aiding in creating products, steering sales teams efficiently, and finding innovative operational solutions daily.
- Digital Transformation Strategies:
- Leveraging Microsoft Azure and Databricks AI frameworks for redefining operations, tracking customer demand, developing new products, and engaging with consumers to deliver customized experiences.
- Automation and Predictive Analytics in Supply Chain:
- Modernized data and cloud infrastructure with automated processes and workflows, applying predictive analytics, AI, robotics, and process automation in business operations to drive supply chain efficiency, predict purchasing trends, and streamline product portfolios.
By The Numbers
- Efficiency: Enhanced accuracy in distribution, driving supply chain efficiency by reducing on-demand forecasting from weeks to days or hours.
More Details
Delve deeper into how PepsiCo is integrating AI in manufacturing and supply chain processes here, here, and here.
Go Deeper
- Consumer Engagement through AI: Explore how PepsiCo uses AI to engage with consumers, understand their preferences, and deliver customized experiences.
- Support for Tech Startups: Discover PepsiCo’s initiative in launching the PepsiCo Lab to support innovative tech startups with solutions for contemporary business challenges.
Industry-Academia Collaborations: Uncover the importance and impact of PepsiCo’s collaboration with the Stanford Institute for Human-Centered Artificial Intelligence on supply chain and manufacturing innovations.
Practical Examples: Amazon Advances Manufacturing and Supply Chain Through Generative AI
Why it matters
Amazon’s AI integration showcases a futuristic approach to manufacturing and supply chain optimization, ensuring agility and efficiency in operations.
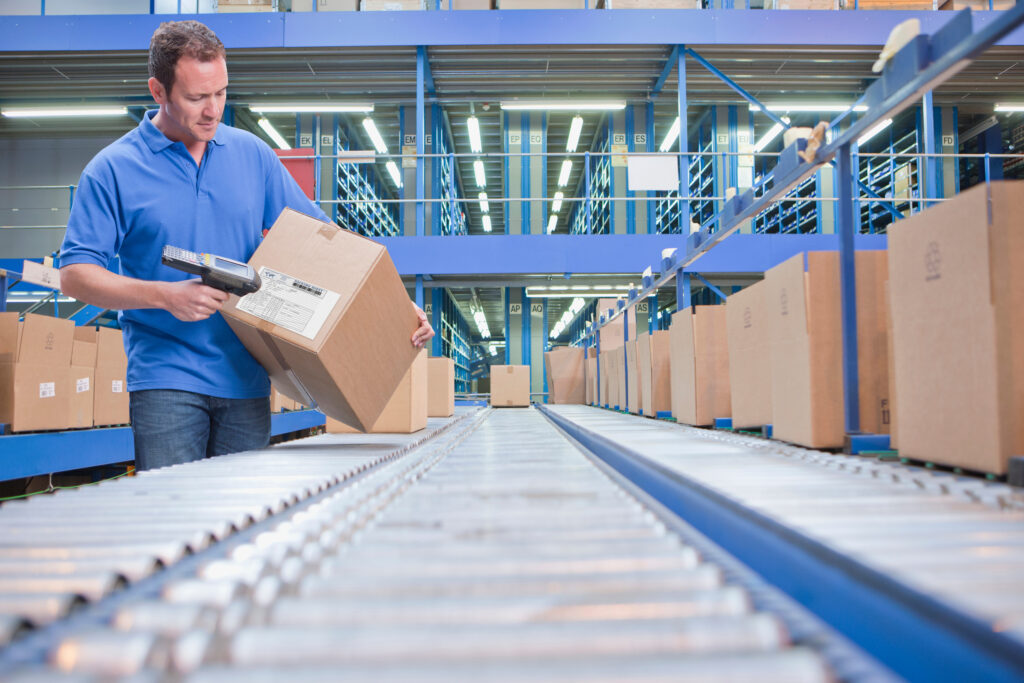
The Big Picture
- Generative AI in Product Engineering:
- Leveraging AI for enhanced design, reducing production time, and exploring manufacturing alternatives.
- Production Optimization:
- Generative AI aiding in capturing and utilizing expert knowledge, potentially maximizing overall equipment effectiveness.
- AI-driven Supply Chain Solutions:
- Addressing supply chain disruptions through AI, reducing operational costs and enhancing brand reputation1.
More Details
Explore Amazon’s perspective on leveraging Generative AI in manufacturing here.
Go Deeper
- Amazon SageMaker in Manufacturing: Discover how startups are employing Amazon SageMaker for AI-enhanced generative design and additive manufacturing.
- AI in Supply Chain Management: Delve into how AI is revolutionizing supply chain management, reducing disruption costs, and improving operational efficiency.
- Amazon Industrial Innovation Fund: Uncover the focus on customer fulfillment, logistics, and supply chain within Amazon’s investment initiatives.
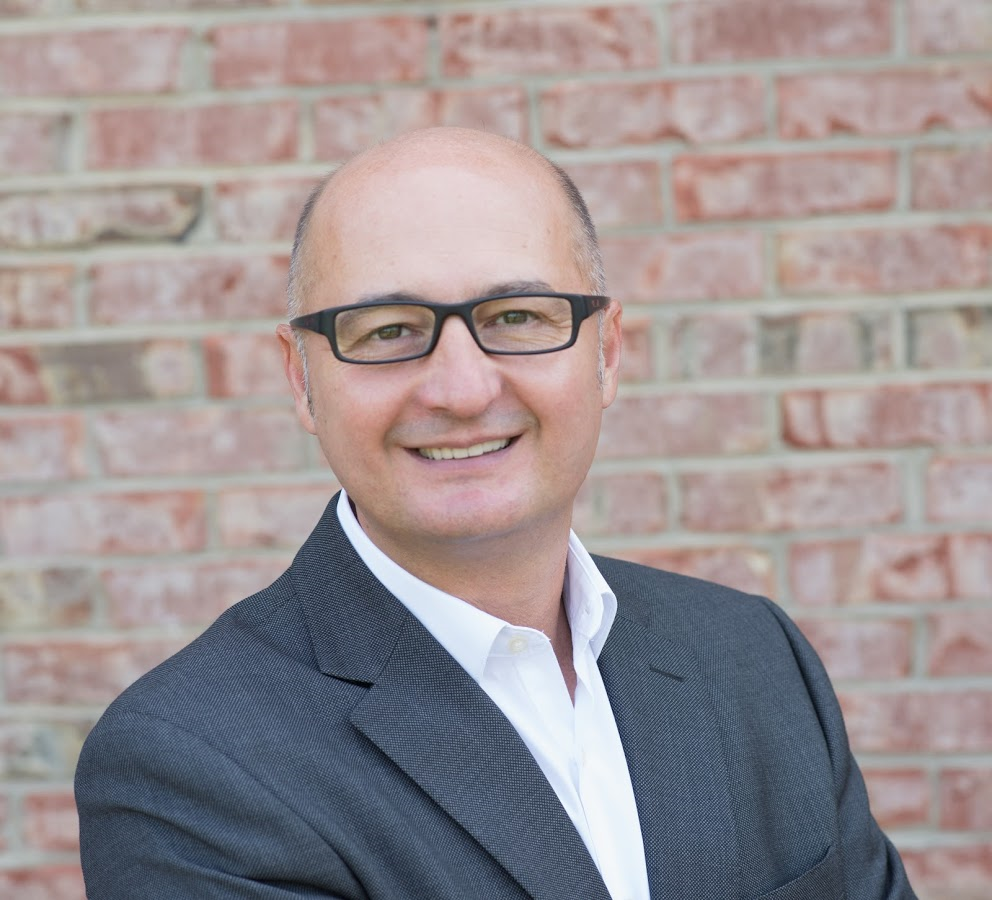
Experienced Machine Learning, Artificial Intelligence, Data Strategy, Information Technology, and Shared Services Executive
Things that matter:
• five largest ML models created at P&G, with over 10,000 pipeline runs/year
• initiated and operated THE data labeling platform and services for ML at P&G, with 300+ projects and millions of annotations on the platform
• Generative AI strategy for the Global Business Units Shared Services for the Brand, R&D, Manufacturing, Supply Chain, Master Data + eComm functions
• Product Management leadership for enterprise-wide Cloud applications combining data and AI
Education
Harvard Business School Executive Education: Artificial Intelligence (Competing in the Age of AI).
Northwestern University: Executive Strategies to Unlock Enterprise Value in Artificial Intelligence
University of Bucharest: Master of Computer Science from the Faculty of Mathematics
CIIM – Master of Business Administration (MBA) with a focus on Finance
Key Certifications:
AWS Certified Machine Learning – Specialty
Azure ML Artificial Intelligence Certification
Data Camp Certified Data Scientist (Python Track)